Design and Development of Machine Learning Model for Antibody Design: Rituximab a Case Study
DOI:
https://doi.org/10.5530/ctbp.2024.3.33Keywords:
Rituximab, Machine Learning, Immunogenicity, Molecular Dynamic SimulationAbstract
The design of antibodies using machine learning has emerged as a cutting-edge approach in the field of medical sciences and therapeutics. This study delves into the principles, methods, and deployment of machine learning approach for antibody design. The present study showed use of extensive antibody databases to train computational models, facilitating the prediction of antibody-antigen interactions. Seven different encoders were used for the vectorization of the antibody and antigen sequences. Conjoint triad showed unsurpassed performance in the machine-learning algorithm with 0.78 correlation and outperformed other encoding methods. The case study of Rituximab was used to demonstrate the practical application of the machine-learning model that was developed. The affinity score predicted by the model was utilised to select the most promising rituximab sequence. Structural investigation employed molecular dynamics (MD) simulation to authenticate the novel sequence (variant) of rituximab. Rituximab variant showed -45.44 kcal/mole compared to the wild type that had -37.66 kcal/mole as a binding free energy for the antigen-antibody complex. Free energy landscape (FEL) was calculated on the first two principal components (PC1 and PC2). The wild type has three minimum energy basins, whereas this variant exhibited only one. This showed that complex produced by antibody variant has a greater capacity to attain global minima. This study sheds light on the innovative application of machine learning in antibody design and also provides compelling evidence of its efficacy through the case study of rituximab. Present study opened new avenues for the development of antibodies with enhanced binding capabilities.
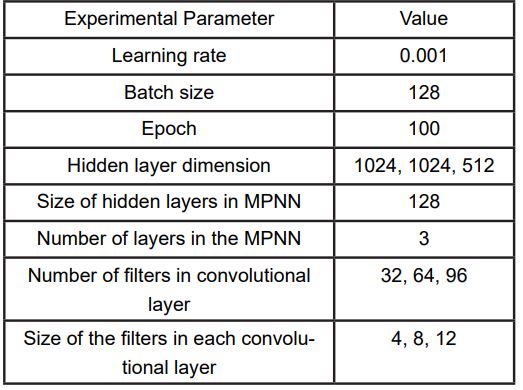